Raise your hand if you’ve been in the following situation.
On second thought, raise your hand if you haven’t been in this situation:
It’s time for the annual business review with senior company executives. The finance team is behind on the year-end accounting, and it’s shutting the window you have to generate business review materials.
Finally, the accounting close is completed and the data is loaded to the data warehouse from which you generate the reports. But wait – there’s a problem: Two of the reports that should match are off by north of $260,000. You can’t brush that one off as a “rounding error.”
What to do?
You’re pretty sure the one with the lowest amount is closer to the truth, but the higher amount will look better to the senior executives. You open a ticket with the BI team to investigate, but you know reverse-engineering the reports and finding the source of the error(s) will take them too long. An hour before the business review, you pick the one with the higher amount.
There’s a variation of Murphy’s Law called the “50-50-90 rule.” If you have a 50-50 chance of getting something right by random guessing, you will be wrong 90% of the time.
Why Traditional Root Cause Analysis Doesn’t Work Anymore
When a report contains errors or, like the example above, two reports that should match fail to do so, the traditional approach to finding the source of the problem is a painstaking manual process. You must examine the report definition and track the path of each item on the report to its original sources. You must account for any calculations, extractions, and transformations along the way.
For a large report in a complex data environment, this process can take weeks. Your business intelligence team has better things to do with their time, but they can’t make any headway because they continually have to stop what they’re doing to chase down reporting errors. The process of finding problems and sending them to the appropriate team member creates a backlog of work that waylays higher priority business intelligence tasks.
With the exponential growth in the volume of business data, not to mention the increasing number and variety of data sources available in the enterprise, the problem of manual root cause analysis can only get worse.
Sick of all the manual mapping required to sort out inconsistencies in your data?
Automated Discovery and Lineage can fix that.
Learn How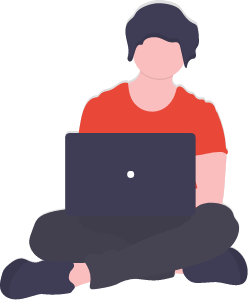
There is a Better Way: Automation
That’s why automation is the better way for BI and analytics. Two important tools can simplify the process of finding reporting errors:
- Automated data discovery
- Automated data lineage
Automated data discovery is the key to BI metadata management. This tool examines all the data assets in your enterprise. It gathers, characterizes, and catalogs the metadata it finds, allowing for faster and more accurate analysis and error reporting.
Automated data discovery enables automated data lineage, which presents a visual map of the path from source-to-report, including ETL and calculation twists and turns the data takes along the way. No more manual parsing through SQL queries and data dictionaries to try to trace the path manually. In moments you can determine where any piece of data originated and the journey it took to its current storage solution.
Together, these metadata management tools make quick work of root cause analysis. Tracking the source of a reporting error can be done in a matter of seconds or minutes rather than days or weeks, and tasks that would have once required the participation of multiple team members can be accomplished by a single data analyst.
Octopai’s automated metadata tools, with their simple, intuitive user interfaces, can free your BI team to do its real job: developing new, insightful reports and innovative dashboards that unlock the value of your data assets.