A data asset is only an asset if you can use it to help your organization. Otherwise, it becomes a liability, taking up space and resources.
What enables you to use all those gigabytes and terabytes of data you’ve collected?
Metadata.
Metadata is the pertinent, practical details about data assets: what they are, what to use them for, what to use them with.
Without metadata, data is just a heap of numbers and letters collecting dust.
Where does metadata come from?
It does not appear ex nihilo or emerge spontaneously out of the ether.
The creation of metadata happens whenever data is created, added to a data organization system or moved. Think of a data asset in the form of a spreadsheet file. Whenever you save the file, you create metadata about the current status of this data asset:
The metadata displayed here includes data asset type, size of the asset, and time of the last change in the asset.
Other useful metadata might include:
- time of asset creation
- name of creator
- definition of the asset
- units of measurement used
- method of computation
- process by which data was obtained
- dimensions, color, compression (for images)
- who is allowed to see or use this data asset
- how you’re allowed to use this data asset
- what other users have done with this asset
- what other data assets this asset is commonly used with
- limitations on or stipulations about the data asset
- what snack people munch while using this data asset (well, maybe not THAT one…)
What is a metadata management tool?
If you have no way of organizing your metadata, it quickly gets added to the data pile up and becomes a liability itself.
To keep your data landscape traversable and understandable, you need a metadata management tool.
A metadata management tool is software that helps organize, categorize and analyze this information about your data assets. Metadata management tools help you understand a data asset’s current status, history, and context, and discover how best to use it for the benefit of your organization.
Metadata Management is a Strategic Data Imperative
Learn why in our white paper which dives deep into the topic
Download the White Paper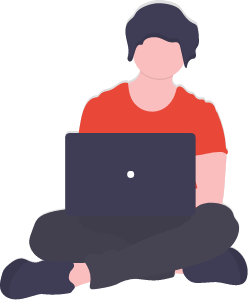
What are examples of metadata management tools?
A drill and a chainsaw are both types of power tools, but they accomplish very different goals. If you try to use a drill to cut down a tree, or a chainsaw to attach a bookshelf to your wall, you’re in for some frustrating times.
Automated metadata management tools are not one-size-fits-all, either.
Let’s take a look at some common enterprise metadata management tool capabilities and their top uses.
Metadata harvesting
How many different data systems do you have in your BI environment? The average data intelligence stack is comprised of the following components – ETL, data warehouse/database, analysis, and reporting – for each of which you may use one or multiple systems. Effective management means centralized management, which means you need to get all your metadata into one place.
Since assumedly you don’t want to take a leisurely stroll through your BI landscape, stopping to smell the roses and plucking out metadata by hand, you’re going to need the equivalent of a metadata combine to do heavy-duty harvesting and processing.
Metadata ingestion and translation
Metadata can be written in so many standards it can make your head spin. Metadata about research projects, for example, can use Dublin Core, RO-Crate, or the Common European Information Research Format.
In order to avoid a Tower of Babel effect when bringing your metadata together, metadata ingestion and translation tools make all your metadata play nice, speak the same language, and refrain from hitting each other over the head with sharp, pointy objects.
Metadata ingestion and translation tools are important for:
- categorizing data
- creating a single source of truth
- reducing redundancies
Metadata repositories
A metadata repository is a tool for the storage and maintenance of metadata. It has to go somewhere, after all!
Business glossaries
A business glossary is an exhaustive list of all metadata terms used across the organization with definitions and how they relate to each other. It is meant to be used by everyone in the organization to eliminate departmental variations on how data is defined, using shared terms that are clearly defined.
Business glossaries are important for:
- reducing redundancies
Data dictionary
A data dictionary contains technical descriptions and detailed information on the files that make up a dataset. Using metadata such as file types, file sizes, and lineage information, data dictionaries organize data so that analysts and data engineers can query and understand the data contained within them.
Data dictionaries are important for:
- categorizing data
- creating a single source of truth
- reducing redundancies
- making data easier to discover for technical users
- evaluating the usefulness of data for a given purpose
Data lineage solutions
A data lineage tool tracks and shows you the history of your data: where your numbers came from, where they ended up, and what happened to them along the way. Ideally, an automated data lineage solution should encompass the in-depth and complete understanding of the data origin, what happens to it, and where it is distributed within your ever-growing data landscape.
Data lineage tools are important for:
- root cause analysis
- impact analysis
- reducing redundancies
- creating a single source of truth
- regulatory compliance
- identifying and understanding relationships in large and complex sets of enterprise data
Metadata stewardship
Ever try to find out who the dirty dish next to the kitchen sink belongs to? Good luck. Even if you can locate likely candidates, rarely does someone want to claim ownership of something that would mean more work for them.
Sometimes you need to find out who is responsible for a piece of metadata: who created it, who updated it, who knows about it. Without metadata stewardship capabilities, your search is liable to run up against a brick wall. With metadata stewardship,, finding the person in charge is as easy as a few clicks (plus they can’t claim it’s not them!).
Metadata stewardship tools are important for:
- Data governance
- Regulatory compliance
- Evaluating and improving data quality
Metadata collaboration
When you want to find the best sushi place in your area, it’s a fair bet that you leverage the knowledge of your community. You ask on your Facebook and WhatsApp groups; you read reviews on Yelp and Google; maybe you text a neighborhood acquaintance you know is crazy about Japanese food.
When you want to find the best data asset to use for a particular purpose, the knowledge of the community is just as valuable. If you can see ratings and reviews of available data assets, learn what other users did with them, and drop a line to a subject matter expert, your chances of choosing great data to use increases a thousandfold.
Metadata collaboration tools are important for:
- improving data quality
- evaluating the usefulness of data for a given purpose
Data catalogs
A data catalog is a tool that organizes all the data assets in a company’s information landscape. Each data asset’s entry in the data catalog includes definitions, descriptions, ratings, roles, and more, making it simple to search for and identify the data you need for any given purpose. A comprehensive data catalog solution often fills many of the integrated metadata management functions of the other tools listed above.
Data catalogs are important for:
- categorizing data
- creating a single source of truth
- data governance
- regulatory compliance
- making data easier to discover for technical and business users
- evaluating the usefulness of data for a given purpose
- identifying, understanding and managing relationships in large and complex sets of enterprise data
Active metadata management tools (AI and machine learning)
Want your metadata to stop lazing about and work for you on its own time? When machine learning and algorithmic analysis enter the picture, finding patterns and anomalies, asking questions and drawing conclusions, metadata management becomes active and intelligent.
An active metadata management strategy can highlight data quality and data privacy issues, correct reports, enrich data science models and point out business opportunities and risks.
Active metadata management tools are important for:
- evaluating and improving data quality
- regulatory compliance
- identifying, understanding and managing relationships in large and complex sets of enterprise data
- data governance automation
Time to fill your toolbox
Implementing metadata management best practices and tools ensures that information can be integrated, accessed, shared, linked, analyzed, and maintained to best effect across your organization.
Make sure your metadata management toolbox is complete, and you’ll be well on the way to turning your data assets from straw into gold.